THE 6TH SYMPOSIUM ON CONFORMAL AND PROBABILISTIC PREDICTION WITH APPLICATIONS (COPA 2017)
14-16 June, 2017
Stockholm, Sweden
Co-organised by: Royal Holloway, University of London, UK and Karolinska Institutet, Sweden
Tutorial Day
13 June, 2017
Location: Widerströmska huset, Karolinska Institutet, Sweden [map]
Program:
Chair: Ola Spjuth
10:00-12:00 | Tutorial 1: Introduction to Conformal Prediction (Henrik Linusson) |
---|---|
12:00-13.30 | Lunch |
13.30-15:00 | Tutorial 2: Conformal prediction in Spark (Marco Capuccini) |
15.30-17:00 | Tutorial 3: Venn Predictors (Paolo Toccaceli) |
The number of participants is limited to 40 attendees, please register via the following link: https://goo.gl/forms/fExruuFGppFWNTuq1.
Tutorial 1: Introduction to Conformal Prediction
Teacher: Henrik Linusson, University of Borås, Sweden
How good is your prediction? In risk-sensitive applications, it is crucial to be able to assess the quality of a prediction, however, traditional classification and regression models don't provide their users with any information regarding prediction trustworthiness. In contrast, conformal classification and regression models associate each of their multi-valued predictions with a measure of statistically valid confidence, and let their users specify a maximal threshold of the model's error rate --- the price to be paid is that predictions made with a higher confidence cover a larger area of the possible output space. This tutorial aims to provide its attendees with the knowledge necessary to implement conformal prediction in their daily data science work, be it research or practice oriented, as well as highlight current research topics on the subject.
Since its development the framework has been combined with many popular techniques, such as Support Vector Machines, k-Nearest Neighbours, Neural Networks, Ridge Regression etc., and has been successfully applied to many challenging real world problems, such as the early detection of ovarian cancer, the classification of leukaemia subtypes, the diagnosis of acute abdominal pain, the assessment of stroke risk, the recognition of hypoxia in electroencephalograms (EEGs), the prediction of plant promoters, the prediction of network traffic demand, the estimation of effort for software projects and the back calculation of non-linear pavement layer moduli. The framework has also been extended to additional problem settings such as semi-supervised learning, anomaly detection, feature selection, outlier detection, change detection in streams and active learning. The aim of this symposium is to serve as a forum for the presentation of new and ongoing work and the exchange of ideas between researchers on any aspect of Conformal Prediction and its applications.
Tutorial 2: Conformal prediction in Spark
Teacher: Marco Capuccini, Department of Pharmaceutical Biosciences, Uppsala University, Sweden
This tutorial will introduce the Spark framework for automation of model building. The Spark framework is a cluster-computing engine for large data processing that makes coding in massively parallel pipelines easy, like never before. Spark applications can be written in Scala, Java, Python and R (we will use Scala in this tutorial), and such applications can run with minor adaption on HPC clusters, cloud computing engines (e.g. Amazon EC2) and local machines. Furthermore, Spark applications are out-of-the-box scalable and fault tolerant. This key features, along with the Spark built-in machine learning library, allows one to code massively parallel pipelines for predictive modeling faster, and they are therefore to be more productive.
Tutorial 3: Venn Predictors
Teacher: Paolo Toccaceli, Royal Holloway University of London, UK
Machine Learning is primarily concerned with producing prediction rules, i.e. functions mapping "objects" onto predicted "labels", given a training set of ("object","label") examples. Practitioners often focus on the value of the prediction, but overlook its uncertainty. Venn Predictors offer a principled way of assigning a probability to predictions, relying on a minimal set of assumptions. One distinguishing feature of Venn Predictors is that they have a theoretically-backed property of calibration, in the sense that the probabilities reflect the long-term distribution. The tutorial will also introduce Venn-ABERS Predictors, which offer an efficient way to transform the output of a scoring classifier into a prediction probability. A comparison with other methods, such as Platt Scaling, will be discussed using a practical example from a Chemoinformatics application.
Sponsors
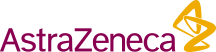
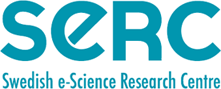