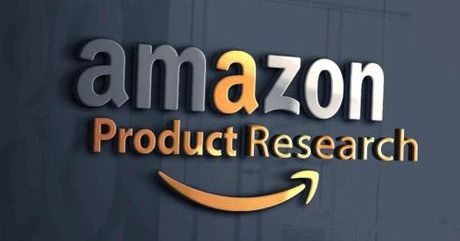
Conformal Martingales for Change-Point Detection
The project was chosen from a competitive field as part of the Amazon Research awards program. The award is worth $90,000 in total, consisting of $70,000 USD in direct funding and an additional $20,000 USD in AWS Promotional Credits.
The project plans to research and apply a novel approach to Change-Point Detection (CPD) - accurate and rapid detection of a change in the distribution of observed values.
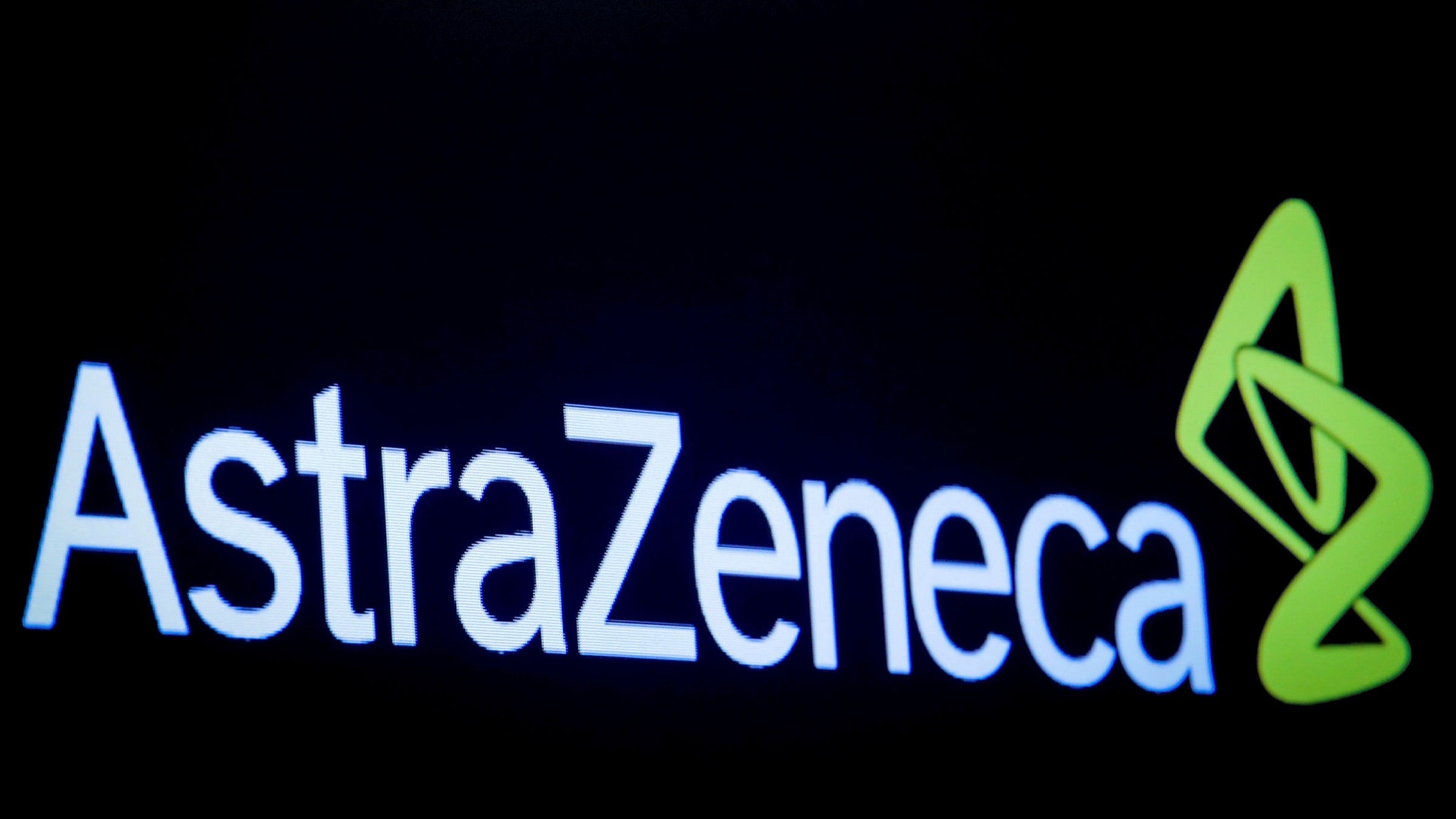
Machine Learning for Chemical Synthesis
The project considers an application of Conformal Predictors to a chemoinformatics problem of identifying activities of chemical compounds. It addresses some specific challenges of this domain: a large number of compounds (training examples), high-dimensionality of feature space, sparseness and a strong class imbalance.
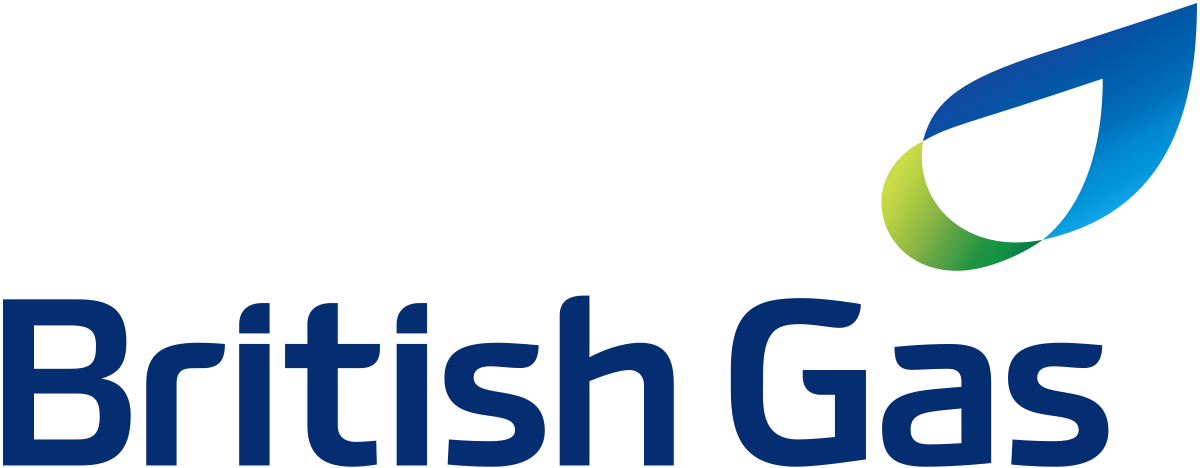
Models of Predictive Maintenance
A new research grant funded by the energy company Centrica will address the problem of predicting the time to the next failure of equipment.
Every year, large sums of money are spent by Centrica on equipment maintenance, including the "rough gas storage" facility. Sensors are located throughout the terminal and measure various physical features of the system, such as temperature, pressure and rotary speed. It is known that the terminal often breaks and that analysis of these sensor readings could provide insight on what caused a given failure and whether another one may occur in a given time frame.
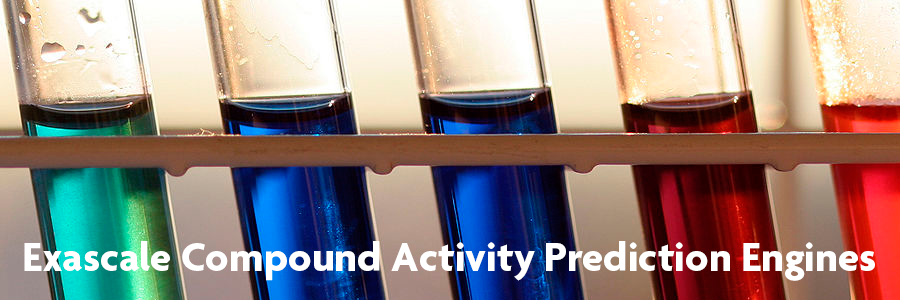
Exascale Compound Activity Prediction Engines
This is part of the €80 billion H2020 European Initiative with funding available over 7 years (2014 to 2020).
We produced state-of-the-art scalable machine learning algorithms with guaranteed performance for future Exascale machines to predict compound bioactivity.
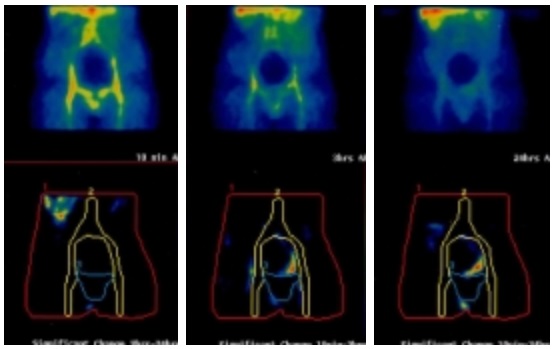
Ovarian Cancer - Adnexal mass categorising
We worked with St. Bartholomew's in London to perform a diagnostic of ovarian cancer, using a variety of symptoms in 287 patients to distinguish benign adnexal masses frommalignant. The above images a patient's scan over a period of 24 hours, with the variation being analysed.
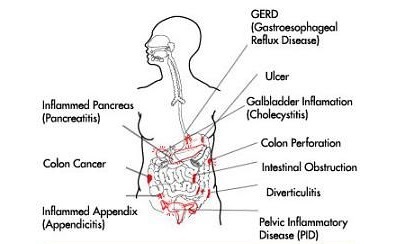
Abdominal pain diagnostic tool
In partnership with Western General Hospital, Edinburgh, we developed a Bayesian algorithm for the diagnosis of abdominal pain in patients.
We analysed data on 6,387 patients, each suffering abdominal pains described by one or more of 33 identified symptoms. Given this data, the learning machine (a G&T system) outputs a probability that the patient was suffering from each of 9 separate diseases.
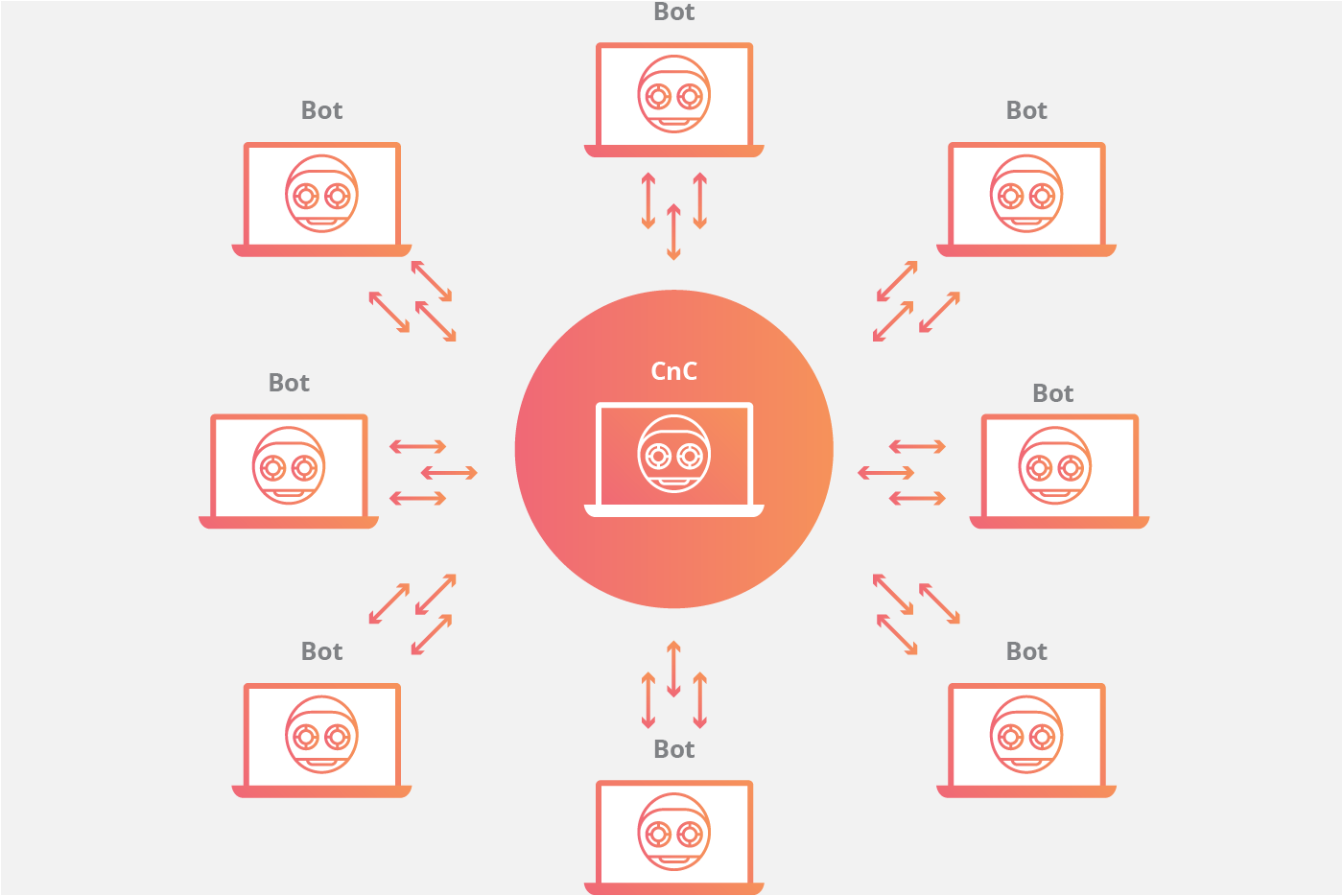
Mining the Network Behaviour of Bots
This project makes cluster-based analysis more accurate and robust against arbitrary obfuscation-based evasion attacks.
In addition, the property of validity can be used to control the number of "alarms" (predicting that a host is compromised) raised by a bot detection algorithm. This is valuable in situations where alarms have to be investigated by human experts but the available manpower is limited.
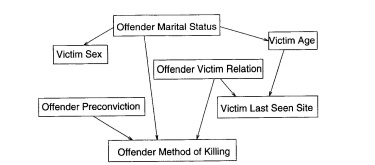
Offender profiling
Using a database set up in 1986 by Derbyshire Constabulary and the input of detectives, we developed an offender profiling algorithm that allowed detectives to input a known variable (eg. Method of killing) to extrapolate further details (probabilities thereof) about the offender.
Such a tool can help focus the limited resources of the investigating detectives. With recent incidents driving a call for improved police databases, such tools could become more prevalent in modern police work.
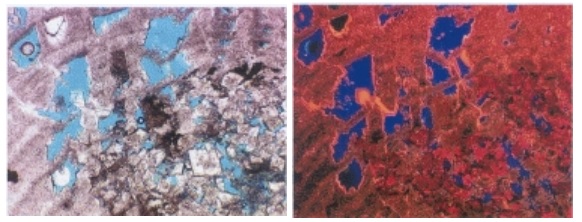
LINK Hydrocarbon Reservoir
We worked with Shell International, Cambridge Carbonates Ltd, British Gas Ltd and Petro-Canada to create a permeability predictor database for use in oil exploration.
The CLRC contributes a learning machine to create a method of comparison, based on analysis of porosity and permeability data, for determining the porosity and permeability of unknown samples.